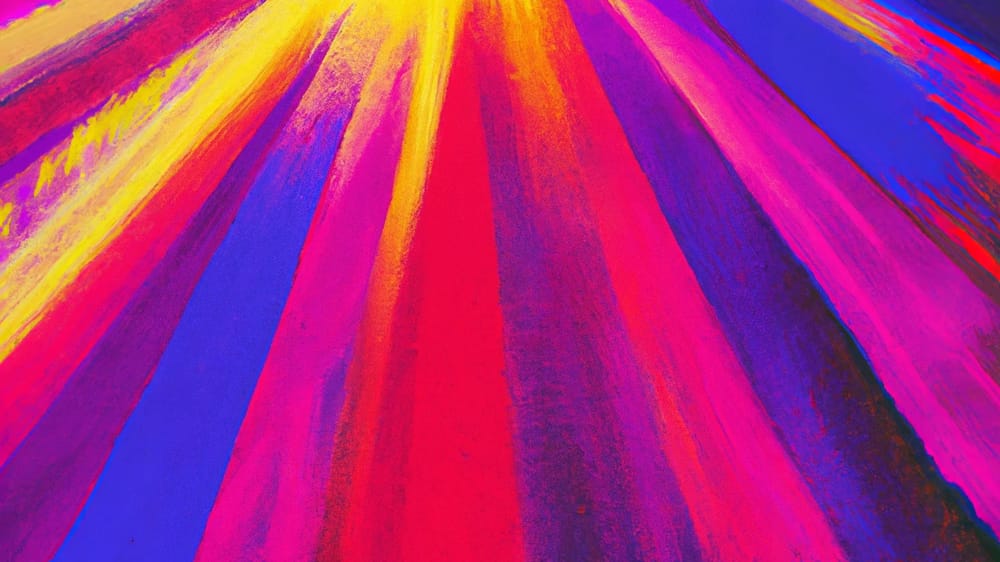
There are still important differences between our current empirical setting and the ultimate problem of fitting superhuman models. For example, it may be easier for future models to mimic weak human errors than for current strong models to mimic current weak model errors, which could make future generalization more difficult.
Nevertheless, we believe that our settings capture some of the key difficulties of aligning future superhuman models, allowing us to begin empirical progress on this problem today. There are many promising directions for future work, including fixing disanalogies in our setup, developing better scalable methods, and advancing our scientific understanding of when and how we can expect good weak-to-strong generalization.
We believe this is an exciting opportunity for the ML research community to make advances in alignment. In order to initiate additional research in this area,
- We are breaking free open source code to make it easier to start working with weak-to-strong generalization experiments today.
- We start a $10 million grant program for graduate students, academics and other researchers to work on superhuman AI alignment in general. We are particularly excited to support research related to weak-to-strong generalization.
Figuring out how to align future superhuman AI systems to be secure has never been more important, and now it's easier than ever to make empirical progress on solving this problem. We are excited to see what discoveries the researchers make.