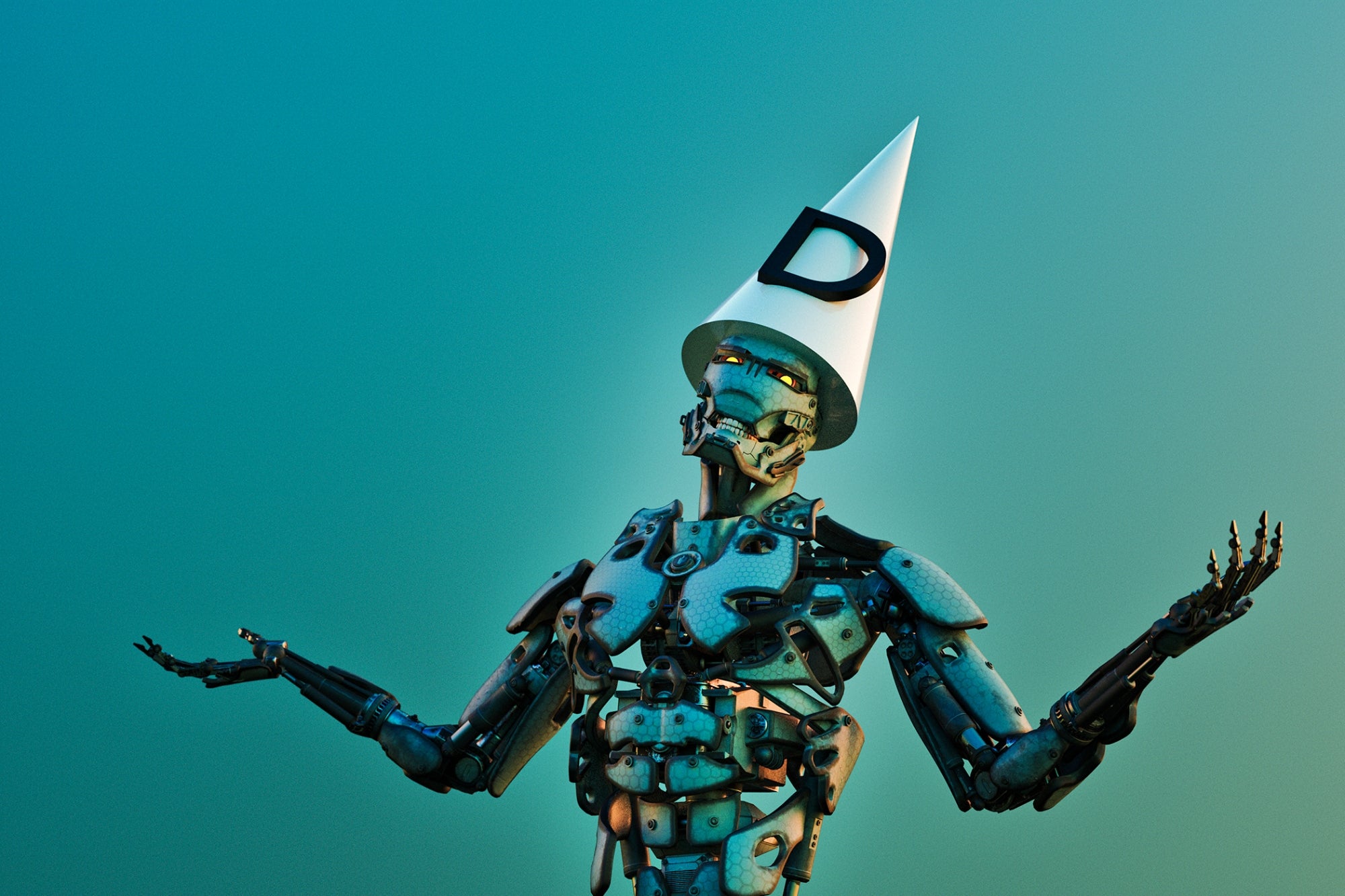
Opinions expressed by Entrepreneur contributors are their own.
Our new AI tools and copilots have made some royal mistakes. They have gave bad advice with high confidence, they have was rumored in dubious dealsthey have made things strange AND became too rude. Of course, mistakes are rare, but when they do happen, the internet goes to town. We like to debunk a bad AI.
But this is a big mistake.
Impulse is partly the result of being threatened from him. But I think it also exposes a deep misunderstanding: we still think of AI agents as machines that aren't capable of real growth and improvement the way human workers are. So we make fun of their mistakes—and point out their mistakes as if they were Roombas stuck in a corner.
In truth, however, we have reached a major inflection point. Today's AI agents are not static. They can grow and learn if we take the time to train them. Moreover, every company already has the power to train its own AI agents.
You don't need a PhD in machine learning. In fact, I've met hundreds of AI agent managers who have never written a line of code. What they I DO know is how people work and how people are best managed. And they understand that these principles now apply to AI agents as well.
Connected: Entrepreneurs are rushing to use AI. Here are the 8 questions you should ask yourself first.
The Golden Rule of People (and AI) Management
The best manager know that human error is a constant, necessary part of human learning. For an employee to truly realize their potential, they must be given the freedom to push the boundaries, experiment and even fail. Expecting a new employee to never waver isn't just unrealistic—it's also counterproductive. Great managers know this clutter and growth go hand by hand.
Meanwhile, exceptional managers know that it's not always the employee who needs to be corrected. It is often the manager's method boarding, training or providing feedback that needs correction. Big companies lose tens of millions of dollars because employees misunderstand policies or processes. However, high-performing managers don't automatically point the finger; instead, they use those mistakes as a jumping off point for introspection and improvement.
The same principles now apply when working with AI agents. They do not come as finished products. Rather (like humans), they need input and a chance to learn for their new jobs. They need feedback. They need it mentoring. In short, managers are finding that AI agents need the same kind of grace already afforded to human employees.
Taking advantage of AI's “teachable moments”.
Say you work at a bank and you're stepping into an AI customer service agent. You've uploaded to the agent every document your human employees use to learn about company policy and procedures (they were read and digested in moments.) Company blogs and changing product details can also be used in AI, simply by provided relevant URLs.
Then, after the AI agent is ready to start working with customers, it finally has a chance to make its first mistake. And you have a chance to improve it.
An explanation of how to open a new checking account, for example, can be too long for customers looking for a quick answer. This is not a fatal flaw. Is one teaching moment. Giving direct feedback—“shorter answers, please”—translates into immediate and visible improvement.
Every response from the agent can be shaped and created, with benefits that add up quickly over time. I've seen managers who invest time in training their AI employees turn an eager “trainee” into a seasoned professional in a matter of months.
The real shift in perspective here comes down to recognizing these agents for what they are: Fallible but eager workers who rarely learn if we give them a chance.
What is gained from AI training has passed her mistakes
The benefits of this change of mind are manifold. In customer service, the large amount of time and money spent on training human agents usually leads to limited returns. Industry-wide, we lose nearly half of those jobs every year. It is a sieve, with company resources flowing down the drain.
In contrast, AI agents aren't going anywhere. Every ounce of effort poured into training an AI agent continues to produce returns in perpetuity. What's more, these returns scale rapidly—a VP at Wealthsimple, a leading online investment platform, recently evaluated that her AI agent delivered to her productivity of ten full-time human agents. This, by the way, allows those people to focus on concierge experiences that are more complex and still require the human touch.
We already know that the quality management of people is directly connected at a higher market cap. Quality management of AI agents promises an even more positive effect. AI agents never forget and never leave, allowing management efforts to be scaled and shared.
But the advantage extends beyond skilled AI agents. Because he NEEDS human management and feedback in order to succeed does not end up just taking jobs – but creating new and often better, those. I've seen frontline customer service employees take on AI management roles, giving them a renewed sense of ownership in the company.
Indeed, managers who learn to train AI agents are making themselves indispensable. They have learned to use a tool that can increase productivity in every other department in their company.
Connected: You Can Fear It and Still Use It – Why Are So Many American Workers AI-Shy?
A future where we are all managers
Nor is this change limited to a few select roles. From here on out, almost everyone will be an AI manager. We will all have AI agents working for us, increasing our productivity. And that means this mind-shift I'm describing—thinking of AI agents as learning and ever-evolving collaborators—will be discussed far beyond the C-suite.
As a new paradigm begins, agents will become as intelligent as we, collectively, strive to make them.
It starts by extending AI agents the same courtesy we extend to humans – understanding that everyone (and every robot) makes mistakes. Then, we do what great managers have always done: train, train, and remove obstacles. They are learning machines, after all, just waiting for the next lesson that allows them to jump forward again. And that's where we come in.