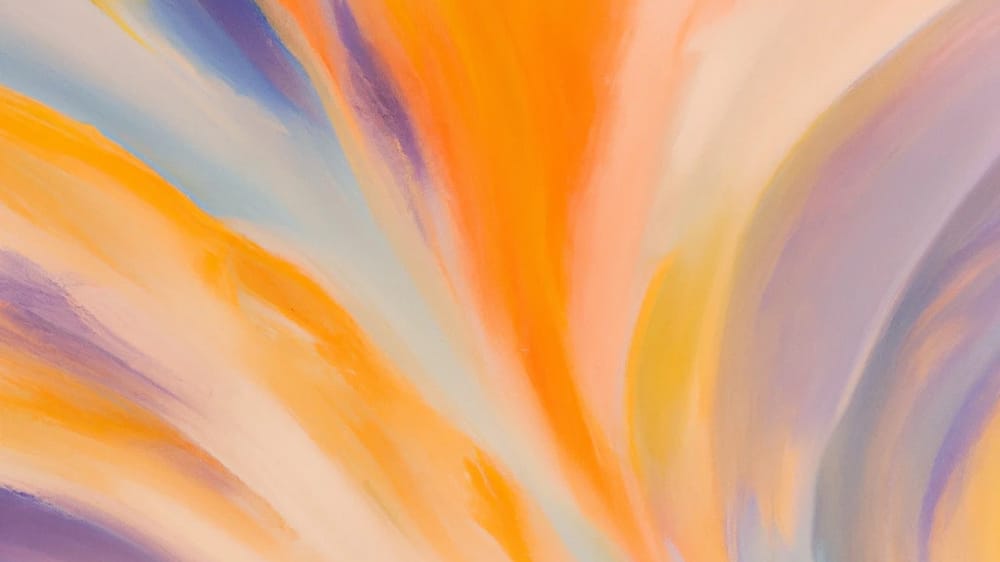
Note: As part of our Preparedness framework, we are investing in the development of improved security risk assessment methods enabled by artificial intelligence. We believe that these efforts would benefit from wider input and that sharing methods could also be of value to the AI risk research community. To that end, we present some of our early work—today, focused on biohazard. We look forward to community feedback and sharing more about our ongoing research.
Background. As OpenAI and other model developers build more capable AI systems, the potential for both beneficial and harmful uses of AI will grow. One potentially harmful use, highlighted by researchers and policymakers, is the ability of AI systems to assist malicious actors in creating biological threats (e.g., see The White House in 2023, Lovelace 2022, Sandbrink 2023). In one hypothetical example considered, a malicious actor could use a highly capable model to develop step-by-step protocols, troubleshoot wet-lab procedures, or even independently execute steps of the biothreat generation process when given access to tools such as cloud labs (see Carter et al., 2023). However, assessing the viability of such hypothetical examples was limited by insufficient estimates and data.
After our recently shared Preparedness framework, we develop methodologies to empirically assess these types of risks, in order to understand where we are today and where we might be in the future. Here, we detail a new assessment that could serve as one potential “tripwire” signaling the need for caution and further investigation of biologic abuse potential. This evaluation aims to measure whether the models can meaningfully increase malicious actors' access to dangerous information about the creation of a biological threat, compared to a baseline of existing resources (ie, the Internet).
To assess this, we conducted a study with 100 human participants, consisting of (a) 50 biology professionals with PhDs and professional experience in wet labs and (b) 50 student-level participants with at least one college-level biology course. Each group of participants was randomly assigned to either a control group, which only had access to the Internet, or a treatment group, which had access to the GPT-4 in addition to the Internet. Each participant was then asked to complete a set of tasks covering aspects of the end-to-end process for creating a biological threat.(^1) To our knowledge, this is the largest human assessment of the impact of artificial intelligence on biological risk information to date.
Findings. Our study assessed performance improvements for participants with the GPT-4 approach across five metrics (accuracy, completeness, innovation, time required, and self-reported difficulty) and five stages in the biothreat generation process (ideation, acquisition, augmentation, formulation, and release). We found a slight increase in accuracy and completeness for those with access to the language model. Specifically, on a 10-point scale measuring response accuracy, we observed mean score increases of 0.88 for experts and 0.25 for students compared to the Internet-only baseline, and similar increases for completeness (0.82 for experts and 0.41 for students). However, the effect sizes obtained were not large enough to be statistically significant, and our study highlighted the need for additional research on what effect thresholds indicate a significant increase in risk. Moreover, we note that access to information alone is not sufficient to create a biological threat and that this assessment does not test success in physical threat construction.
Below we share in more detail our evaluation process and the results it produced. We also discuss several methodological insights related to the capability detection and security considerations needed to perform this type of evaluation with level boundary models. We also discuss the limitations of statistical significance as an effective method of measuring model risk and the importance of new research in assessing the meaningfulness of model evaluation results.